.jpg)
Customer churn prediction is hard — we get it. Especially when you consider most customers don't tell you when they're unhappy.
According to a study conducted by Customer Strategy Consultant Esteban Klosky, only 1 out of every 26 troubled customers files a complaint. That means almost 96% of the time you have to rely on something other than direct feedback to identify a churn risk. The odds are not in your favor.

That's why we in B2B SaaS use customer churn prediction to find the silent majority of unhappy customers.
Churn prediction uses data to forecast when a customer might stop doing business with you. It's a way of analyzing current customer behavior and comparing it to past trends so you can anticipate future actions. If you know that customers who stop using the chat feature in your platform are likely to churn, you can watch for that behavior and jump in to save a customer when it happens.
Unsurprisingly, predicting churn using this method is a delicate balance, which takes time and experience. Like any learned skill, there are plenty of mistakes to make as you figure it out.
No fear. We've watched hundreds of customer success managers (CSMs) navigate churn prediction and have found a few common mistakes.
The good news? Knowledge is power here. Buckle up as we show you how to avoid making the five most common customer churn prediction mistakes we see most often.
Customer Churn Prediction Mistake #1: Not tracking the product champion
Product champion (n.)
The most influential user of your product. They either made the buying decision or heavily influenced the person who did.
Not tracking the actions of a product champion can lead to a false reading of customer health and a missed chance to ID a churn risk.
Let’s say your customer - Hulu - has 500 employees using your product. Most of those users are engaging with your platform regularly, enabling all the features, and providing positive feedback. Hulu seems like a healthy customer, right?
But the product champion at Hulu hasn’t used your software in weeks. Plus, they just turned off three integrations. Could they be trialing your competitor’s software? You’d never know because that one user’s actions are barely a blip in Hulu’s overall analytics.
The solution:
Track the behavior of the product champion separately from the overall account. The criteria you use to track the product champion is up to you. It might be as simple as how often they engage with your product. Or it could be a custom metric you’ve created.
- Make sure you've implemented proper product usage tracking, perhaps using a tool like Segment.
- Tag champions with a trait so you can separate them from the pack
- Set up some dashboards and/or alerts in your Customer Success Platform focused on identifying accounts with unengaged champions.

Customer Churn Prediction Mistake #2: Prioritizing product usage over implementation during onboarding
Low engagement with key features is a good indication that a customer is going to churn—if people aren’t using your product, why would they pay for it? It's why we usually see customer health scores that weigh product usage heavily.
But it’s a different story during the onboarding phase. When a customer is onboarding, the focus is on actions required for setup, not your differentiating features. Not performing these actions during onboarding is a clear churn risk sign, regardless of how many people are actually using the product.
That's why product implementation is a better indicator of account health than product usage during the onboarding phase.
The solution:
Use different health scores for users who are onboarding
- Organize your accounts into separate segments/stages based on their lifecycle
- Define health scores that favor different actions for each segment. Weigh implementation actions heavily for those in onboarding and recurring/differentiating actions heavily for those who are onboarded.
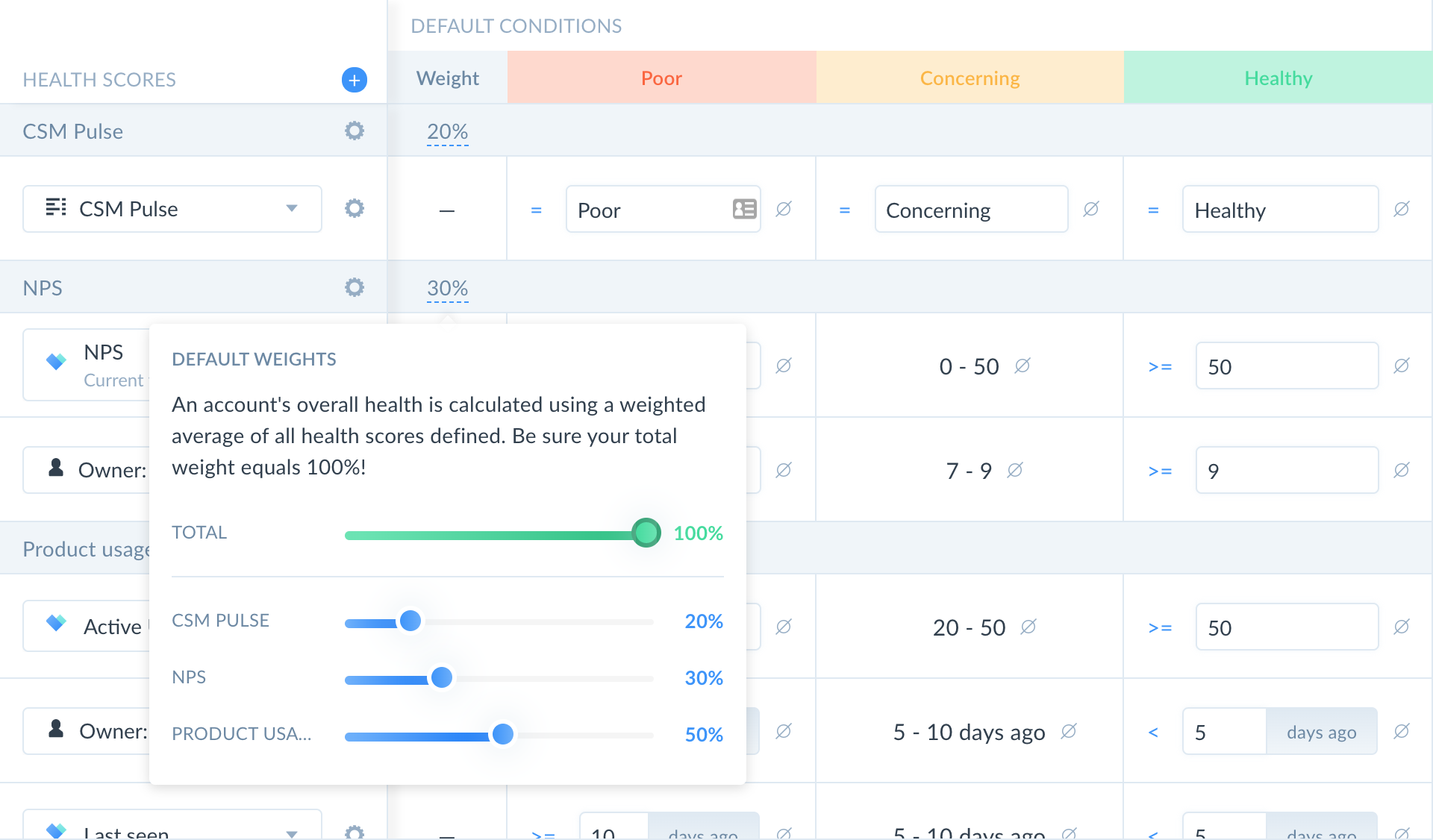
All of your new customers will be tracked by the onboarding health score until they’re fully set up in your product. Then, you can move those accounts to a standard health score that emphasizes factors like product usage or NPS score.
Want modern health scores?
Learn how to automatically segment your customers and define different health equations for each segment
Customer Churn Prediction Mistake #3: Not tracking integrations as they’re disabled
A user enabling an integration is a reason to celebrate. It means they’ll get more value out of your product. But what does it mean when your customer disables an integration?
At Vitally, we think of disabling an integration as a potential churn signal. For example, Vitally offers an integration with Salesforce. If one of our customers disables that integration, it could mean they’re trialing another customer success platform - one that also integrates with Salesforce. We would never notice that churn signal if we only tracked integrations as they’re turned on.
The solution:
Every time you set up a new integration, make sure to track both when a customer enables it and when they disable it (which is hopefully never).
- Track a product event when an integration is enabled or disabled
- Build a dashboard in your Customer Success tool that 'ties' the enabling and disabling events together
- Look to see where the integration is currently—on, off, or used to be on but is now off
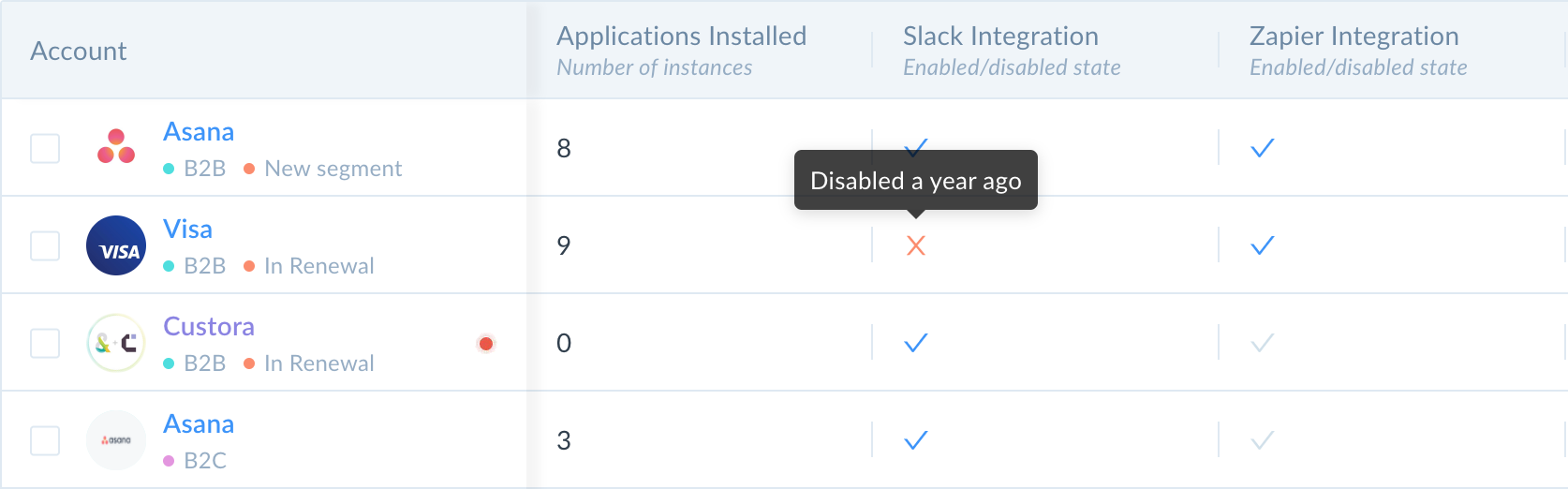
Bonus: Track when an account admin disables an integration
Those users are typically the first to make a change, and disabling an integration is a signal that a change could be happening.
Customer Churn Prediction Mistake #4: Not normalizing usage rate for population
Population (n.)
The number of people using your product at a given company. Sometimes referred to as “seats” or licenses.
To normalize the usage rate for population, divide the number of times all the users on an account use your product in a meaningful way by the total number of people using it at that company.
If you don’t normalize for population, you’ll incorrectly interpret the health of a user and miss a potential churn signal.
Let’s say you’re a CSM at Asana, a web-and mobile-based app that helps teams organize, track, and manage their work. In an ideal world, every user is creating and completing tasks. If some number of people aren't, it could indicate a churn risk.
But what is that number? For Asana, a customer could mean one lone consultant or a massive team like Salesforce that has hundreds of Asana users. If you—an Asana CSM—focused on the number of tasks created per account, the numbers would be nonsensical. A lone consultant who creates 10 tasks in a week is great. But if all of Salesforce created only 10 tasks in a week, that would be a huge problem.
The solution:
Normalize your usage rate to create data sets that have meaning.
- Start by tracking critical interactions with your product (Asana might track each time a task is created and who creates it)
- Track the number of users on an account
- In your Customer Success tool, set up a metric that divides the number of occurrences of the critical action (#1) by each account's user population (#2) to get a normalized metric

Let's take the Asana example from above. Salesforce has 100 users. In total, they use Asana 500 times in one day. That means the daily usage rate, normalized for population, is 5.

Ideally, you'd automate this calculation and output it somewhere your CSMs can take action on it.
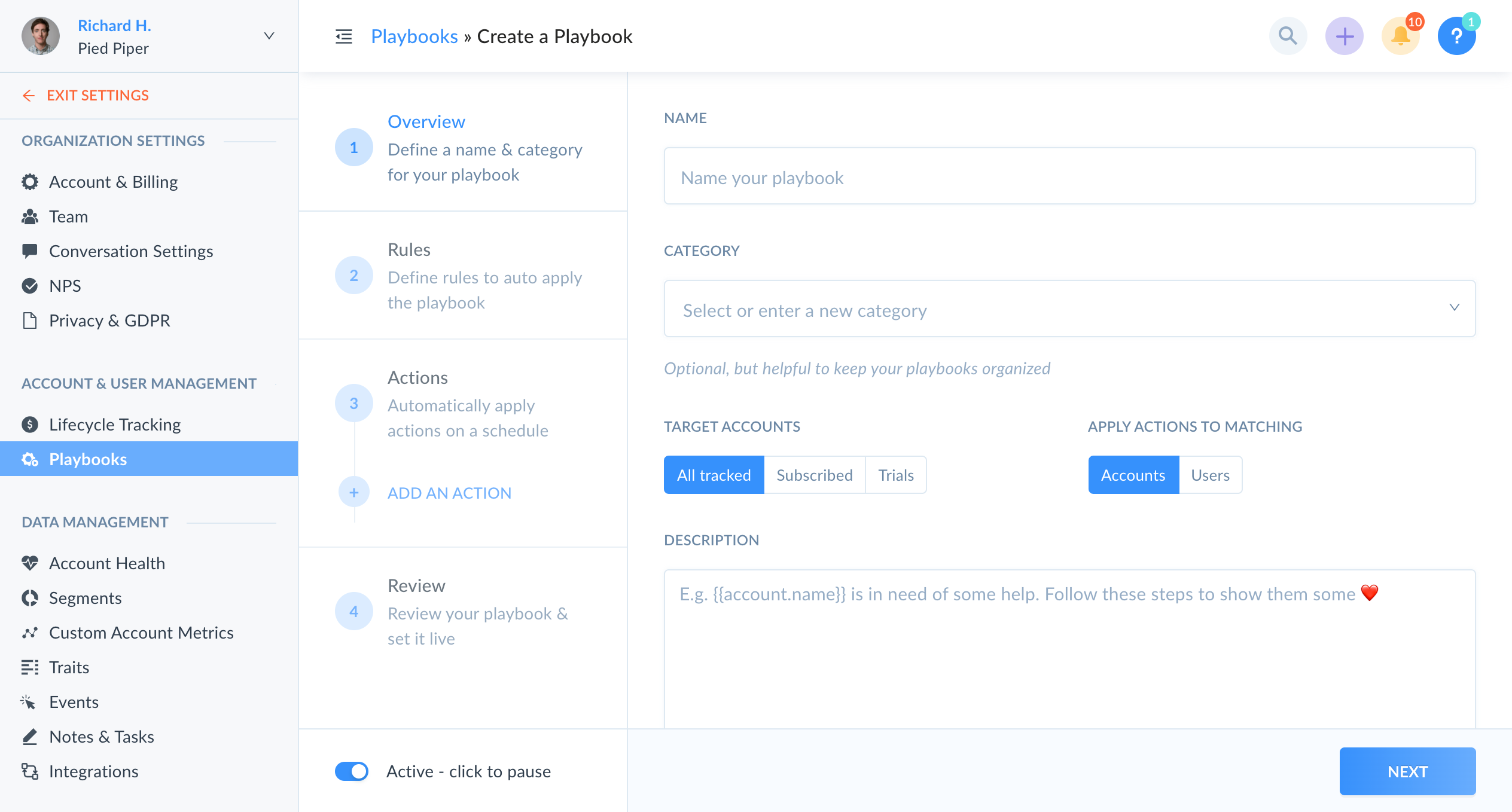
How much a customer uses your product directly correlates to customer health. The more they use it, the more value they’re getting out of it. But you can’t just look at today’s usage. You need to compare it to the trend over time to know whether a customer is healthy or a churn risk.
Customer Churn Prediction Mistake #5: Considering the current state of customer health, not the change over time
No matter what success metric you’re looking at, the real story is in the trend, not the current state.
Let’s say your team has agreed that any customer health score over 7.5 is healthy. Today, your customer has a health score of 7.8. They're healthy, so no action needed, right? Not so fast—last month, their health score was 8.5. Why the slip?
You’ll never think to ask that question if you're only looking at only the current state and not the trend.
The solution:
There's really no way around this one—you just have to track trends over time. You can do it with a spreadsheet or an automatic tool, but it's especially helpful if you have it in a setup where you can trigger automatic actions against it.
- Set up custom metrics and/or health scores in your Customer Success tool, making sure you have a way to track them historically
- Build automated workflows across both the current value and a historical value
- Alert your team of accounts that dip by some threshold over some timeframe (e.g. health score dips by 10% over any 7 day window)
Bonus points:
Take an action when a customer is trending up in a given category. Those customers are ripe for upsell. They’re also the ideal type of customer your sales team should be targeting.
Customer Churn Prediction leads to increased profitability
A study by For Entrepreneurs showed that it costs SaaS businesses nearly 9X more to acquire a new customer than it does to retain an existing one. Research by Frederick Reichheld of Bain and Company revealed that just a 5% increase in customer retention increased profits by 25%.
The message is pretty clear: The road to SaaS profitability is shorter for startups that focus on reducing customer churn.
It would be great if customers told us they were thinking of leaving. The vast majority of the time, they don’t. So if you want to lead your SaaS startup down the shorter road to profitability, you’ll need to get good at predicting when a customer might churn. Overcoming these five customer churn prediction mistakes will put you well on your way.